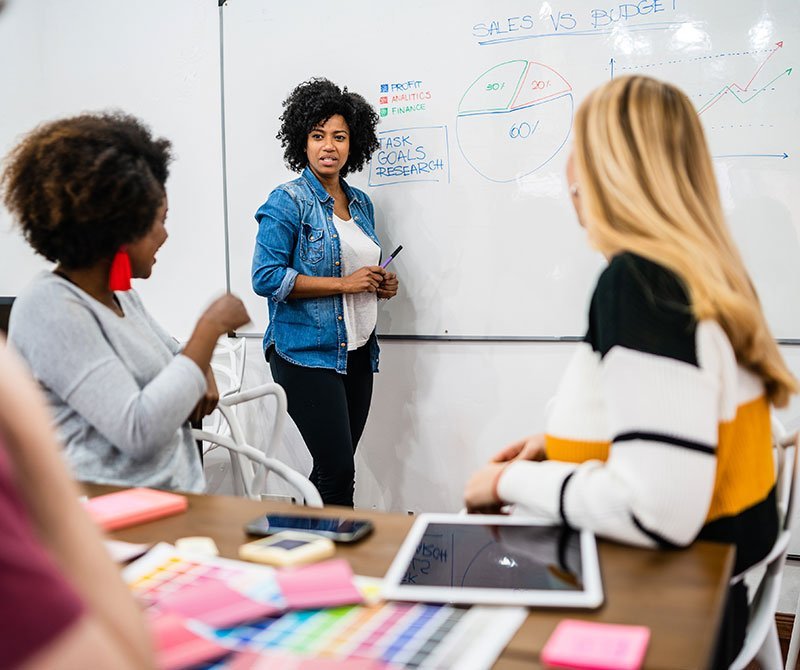
AI/ML
We are a leading product and services company and often tailor best practices to the specific needs and challenges of clients. By following our guidelines, organizations can leverage AI/ML to drive innovation, improve operations, and achieve their business goals.We often provide guidance and best practices for implementing AI and machine learning (AI/ML) in various industries. While specific practices can vary depending on the context, our best practices are highly recommended due to following guidelines.
Business Alignment:
Start with a clear understanding of the business problem or opportunity that AI/ML can address.Align AI/ML initiatives with overall business strategy and objectives.
Proof of Concept (PoC):
Start with a small PoC to validate the feasibility and potential benefits of AI/ML before full-scale implementation.
Data Quality and Governance:
Ensure high-quality data, as the success of AI/ML projects heavily depends on the quality of the input data.
Implement data governance practices to maintain data accuracy, privacy, and security.
Model Selection and Evaluation:
Choose the appropriate machine learning algorithms and models based on the problem and data.Evaluate model performance using metrics relevant to the business problem, and iterate to improve performance.
Ethical Considerations:
Address ethical concerns such as bias, fairness, and transparency in AI/ML models and data.Implement responsible AI practices to ensure that AI applications adhere to ethical standards.
Scalability and Deployment:
Design AI/ML solutions with scalability in mind to handle increasing data volumes and user loads.Develop a strategy for deploying models into production environments effectively.
Interdisciplinary Teams:
Assemble cross-functional teams with expertise in data science, engineering, domain knowledge, and project management.
Foster collaboration between data scientists and domain experts.
Iterative Development:
Adopt an agile approach to AI/ML development, allowing for quick iterations and improvements based on feedback.
User-Centric Design:
Prioritize user experience by designing AI solutions that are intuitive, user-friendly, and aligned with user needs.
Monitoring and Maintenance:
Implement monitoring tools to track model performance, detect drift, and ensure models remain effective over time.Regularly update and maintain models to adapt to changing data and business conditions.
Data Privacy and Compliance:
Comply with data privacy regulations (e.g., GDPR, HIPAA) and implement robust security measures to protect sensitive data.
Education and Training:
Invest in training and upskilling employees to understand AI/ML concepts and their implications in the business.
Cost Management:
Continuously monitor and manage AI/ML project costs to ensure that they align with budgetary constraints.
Change Management:
Prepare employees for changes resulting from AI/ML implementations through effective change management strategies.
Communication:
Maintain clear and transparent communication with stakeholders about project progress, challenges, and outcomes.
Partnerships:
Collaborate with technology platform providers, open sources, or academic institutions to access cutting-edge AI/ML solutions and expertise.
Long-term Strategy:
Develop a long-term AI/ML strategy that evolves with changing technology and business needs.