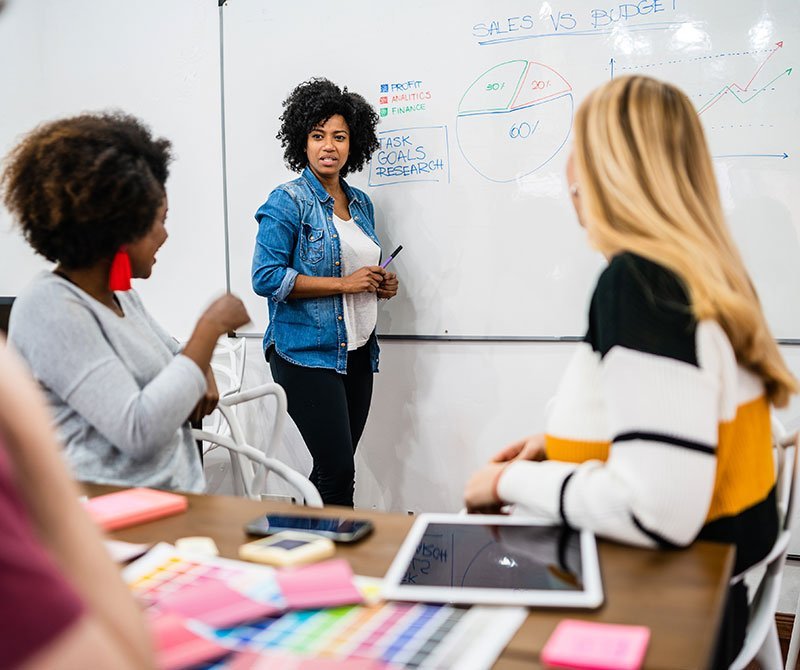
Data and Analytics
Please note that industry standards and Data Analytics trends are changing rapidly, and we have been able to stay updated with the latest developments in data analytics by leveraging industry publications, attending conferences, and networking with professionals in the field. In terms of data analytics, specific trends and standards may vary by industry, so it’s important to consider the unique requirements of client’s business sector.
Advanced Analytics and AI/ML Integration:
The integration of advanced analytics, machine learning (ML), and artificial intelligence (AI) into data analytics is a prevailing trend. Organizations are leveraging predictive and prescriptive analytics for data-driven decision-making.
Big Data and Real-time Analytics:
With the increasing volume of data generated, big data analytics and real-time data processing have become more prevalent. Technologies like Apache Spark and Kafka are commonly used for real-time data analytics.
Data Governance and Compliance:
Data governance and compliance with regulations such as GDPR and CCPA remain high priorities. Organizations are focusing on data quality, security, and privacy.
Self-Service Analytics:
Self-service analytics tools and platforms are empowering non-technical users to perform data analysis independently. These tools are designed to be user-friendly and require minimal coding skills.
Cloud-based Analytics:
Cloud computing platforms like AWS, Azure, and Google Cloud are increasingly popular for data storage, processing, and analytics. These platforms offer scalability, flexibility, and cost-efficiency.
Data Visualization:
Effective data visualization tools and techniques are crucial for presenting data insights to non-technical stakeholders. Dashboards and interactive reports are commonly used for this purpose.
Augmented Analytics:
Augmented analytics involves using AI and ML to enhance data analytics processes. It can assist in data preparation, insight discovery, and natural language generation for reports.
Data Science and Data Engineering Collaboration:
Close collaboration between data scientists and data engineers is becoming standard practice. Data engineers prepare and transform data for data scientists to use in modeling and analysis.
Explainable AI
The industry is placing a greater emphasis on making AI and ML models more interpretable and transparent, addressing concerns about bias and ethics in AI.
Edge Analytics:
Edge analytics is growing in importance, especially in IoT and industrial applications. It involves processing data at the edge of the network, closer to the data source, to reduce latency and enable real-time decision-making.
Predictive Maintenance and Anomaly Detection:
Predictive maintenance and anomaly detection using AI/ML are widely adopted in industries like manufacturing and healthcare to prevent equipment failures and improve operational efficiency.
Natural Language Processing (NLP), Large Language Models, Generative AI:
NLP is used for sentiment analysis, chatbots, and text mining. It plays a significant role in analyzing unstructured text data, such as customer reviews and social media content.
Data Literacy Programs:
Organizations are investing in data literacy programs to educate employees about data concepts, tools, and best practices, enabling more informed decision-making across all levels of the organization.